“The world is facing a public health emergency caused by the COVID-19 pandemic. We all need to take this on.” – The ELLIS Society
All the scholars, researchers, scientists, and organizations around the world are doing everything they can to fight against the present viral illness that has engulfed the earth. These efforts range from working towards vaccines and treatments all the way to spreading awareness about this disease in the best possible ways. Keeping in mind the concept of social distancing, virtual conferences and workshops are an excellent way to talk about various aspects of this pandemic and its potential interdisciplinary and multidisciplinary combating strategies. One such gathering was organized by the ELLIS society recently.
The mission of the ELLIS (European Laboratory for Learning and Intelligent Systems) society is the creation of a diverse network that promotes research excellence and advances breakthroughs in AI, to educate the next generation of researchers. In the wake of the current coronavirus disease, this society held an online workshop on 1st April 2020. The aim of this virtual gathering was the discussion of various projects to tackle COVID-19 using methods of artificial intelligence (AI) and machine learning (ML), undertaken by the leading international researchers. The research topics included epidemiological modeling, outbreak prediction, drug development, and health care management, to name a few.
The workshop started with a welcome address from Bernhard Schölkopf (Director, Empirical Inference Department) and Oliver Stegle (Leader, Stegle Research Group – Statistical genomics and systems genetics). They highlighted that the role of the ELLIS Health Program is to foster cutting-edge AI/ML techniques to exploit high-throughput data in biomedicine.
Data Science Against COVID-19
Nuria Oliver, a computer scientist well known for her work in computational models of human behavior, mobile computing, human-computer interactions, intelligent user interfaces, and big data for social good, delivered the first lecture of this online workshop. Titled ‘Data Science to fight against COVID-19,’ it emphasized better decision making during the current pandemic through proper analysis of data.
“I have a lot of experience working on the use of data for infectious diseases. For many years, we have experienced a number of barriers that prevent these technologies to be utilized in practical terms,” she said. One of the main realities of the COVID-19 crisis is that decisions are being made without proper and adequate evidence. The first reason for the same is that in public administrations, there are a lot of barriers related to capacity, awareness, digital mindset, and necessary skill sets. There are also some concerns about data protection and privacy. Another problem is the gap between the research and policymaking communities. Then comes the issue of lack of preparedness for immediate and organized actions during such times of need. “To overcome all these limitations, we have created a data science organization that is working directly with the President of the region with different teams,” she explained.
These teams constitute mobile data analysis (mobile networks and apps), epidemiological models (building predictions on the number of infections with different levels of granularity and involves metapopulation and compartment models) and additional modeling. The mobile group is trying to understand questions like how has mobility changed because of contention measures, have the intervention services impacted mobility, and what type has dropped, in the long list of many others. Similarly, the epidemiological group is addressing issues such as the number of infected people under different mobility scenarios, whether the social contention measures are sufficient and the main causes of infection. The additional modeling group is involved with predictions of death, intensive care, hotspot detection and looking for new data sources that would help in making better decisions.
Still, there is a lack of relevant data such as that related to the social contact behavior of people during this contention, economic and labor impact of the COVID-19 crisis on their lives, the prevalence of symptoms in the population, and the lack of tests – the million-dollar question is that how many people are really infected? A few conclusions from this project include the dire need to involve citizens in getting data, the importance of gender and age, and urgent implementation of quarantine infrastructure and more tests.
Genome Analysis Explores COVID-19
Next in line, was the talk by Richard Neher (Head of the pathogen evolution, genomics, and biophysics research group at the Biozentrum, University of Basel, Switzerland), titled ‘Tracking SARS-Cov-2 using viral genomes and projecting its spread.’ Talking about one of his projects, Nextstrain, he explained that it tracks the genome and genetic diversity of a pathogen and outbreak. It was started five years ago, mainly to study the evolution of influenza – this is the virus that continuously circulates and we need to update the vaccines frequently and hence, it is very important to have an up to date picture of its genetic diversity. With the increase in the COVID-19 infection rates, the researchers set up a Nextstrain to understand it more.
On observing the phylogenetic trees, it is seen that the virus is accumulating mutations (modifications in its genes) over time – these are mostly random copying mistakes that the virus replication machinery makes. These representations also provide an idea about the geographical diversity of this illness and how it has spread across the world in these past few months. So, basically, these genomes are providing us the outbreak structures and the scientists expect some changes every two weeks.
This data got developed into a web app that was utilized as an idea to plan hospital capacities. It allows us to specify a particular population with an age distribution (age is crucial because old people tend to get severely affected by this pandemic) and how different mitigation measures can reduce the spread. It gives a model output overlaid with the actual observed cases, which is meant to give some crude idea of how the information we have, when put in place, might conspire to generate different future dynamics. Of course, there are uncertainties in terms of issues related to an inadequate number of testing, and so on.
Microbial Monitoring Network Combats COVID-19
Christopher E. Mason, Head of the Mason laboratory (Weill Cornell Medicine) that works on a ten-phase, 500-year plan for the survival of the human species on Earth, in space, and on other planets, delivered a thought-provoking session titled ‘A Rapid, International COVID-19 and Microbial Monitoring Network.’
This work is focused on finding the evidence of the virus in the environment as compared to inside the patients. The group wants to build a global map with the idea that if this had been done routinely, we might have been able to track the emergence of different RNA viruses. The predictive microbial signatures can predict what city a person is from and the emergence of the virus in different cities (some more than others) with about 88% of accuracy.
Since the past few months, pilot sampling has been going on across 59 cities in 25 countries of the six continents (about 10000 locations) around the world, to track the coronavirus in different environments. One interesting data observed was that in the public services approximately 10% RNA was human, 1-2% was viral and 30% was bacteria, and this finding is pretty new. A couple of wet-lab experiments are aiding in identifying the presence or absence of this virus in the environment.
The group is trying to set up Pop-up Labs across New York City to make it easy to do experiments such as the Loop-mediated isothermal amplification (LAMP) assay. An interesting way is to ask for people’s phones, do the sequencing, and get an estimate of the microbes – earlier it was just for fun but now it is for clinical purposes! The long term dream is to look at the genetic variations, differential expressions in the host cells, host immune cell diversity, changes in the RNA functions and anything else that is there (bacteria and viruses). These are a few things that can be done on the computational side with these samples from the host and microbial perspective. Moreover, COVID-19 Host Genetics Initiative was also started by the group recently and is basically a place where people are looking at the host susceptibility and sharing data from genome sequencing to gain some new insights.
Speedy Drug Development Is The Need Of The Hour
This section housed two lectures by Guenter Klambauer (Institute for Machine Learning) and Yoshua Bengio (one of the world’s leading AI and deep learning experts) respectively.
There are different scales to approach this type of crisis viz., the population scale (epidemiological modeling, etc.), macroscopic scale (healthcare management, patient surveillance), microscopic scale (diagnosis) and molecular scale (drug development, etc.). Titled ‘Screening for SARS-CoV inhibitors,’ the first talk by Dr. Klambauer focused on approaching the coronavirus crisis at the molecular level – the drug development stage.
There are multiple ways to approach the drug development aspect. One of the basic motives is to design a molecule that can inhibit or hamper the virus at different levels. For example, breaking some of its proteins or inhibiting its replication machinery. In present times, computer-aided drug discovery is prevalent. Almost all the stages of this approach are greatly influenced by machine learning.
The very first step is compound designing and ML has been gaining popularity in this field since 2016 to generate molecules utilizing VAR, GAN, RL, RNN and hybrid approaches. It can be a bit faster if one does virtual screening which is the second step. It is basically done using a computer model to screen through a large database of molecules that are already available. For this, there are three principal techniques which include ligand-based, structure-based and hybrid approaches.
Typically, a drug discovery pipeline has a very long time scale. One starts with millions of compounds, then with the help of a virtual screening selects a library of about 10000 compounds. These are slowly reduced to a couple of favorable candidates over time. The next steps comprise the pre-clinical and clinical trials. Finally, it goes to approval from the FDA and if lucky we get the medicine. Given the current emergency, there is a dire need to speed up this entire process and machine learning can help a lot in this case. ML helps us to select very good molecules at the beginning (by predicting parameters such as side effects and efficacy) which in turn makes us faster. There is a new wave of computational drug discovery with Deep Learning. For example, Deep Neural Networks are pretty good at predicting multiple toxic effects at once. For some of the drug targets, the predictive quality is so high that it yields virtual wet-lab tests/assays. Similar large scale virtual screening is being applied to get SARS-CoV2 inhibitors. The scientists have also screened the DrugBank database with this model and come up with the ranking of molecules that are already in the market and hence, can be used in the process of drug repurposing.
In the session ‘COVID-19 research at MILA,’ Dr. Bengio talked about two aspects, drug discovery, and contact tracing and risk estimation. These are among the several projects at the Montreal Institute for Learning Algorithms that have sprung into action in the wake of the novel coronavirus disease. This pandemic has brought solidarity in the science community in terms of how it is fighting with this crisis – through global collaborations, focused on saving lives.
Digital contact tracing using phones by interviewing people about whom they met can have a significant impact on limiting the spread of this pandemic. MIT Media lab and MILA are developing apps for this purpose in which ML methods produce a more accurate prediction of risk. One of the things of emphasis during this process is the privacy of users. Privacy issues range from the development of stigma and subsequent violation of human rights all the way to spreading panic if information goes in the wrong hands. The immediate solution is to start by deploying an app which is very strong in terms of privacy and analyze how far we get in limiting the rate of the infection of the virus. There is a need to balance these conflicting terms – privacy and health protection – to achieve effective results. The researchers are working on the private set intersection method coupled with ML tailored to the COVID-19 situation.
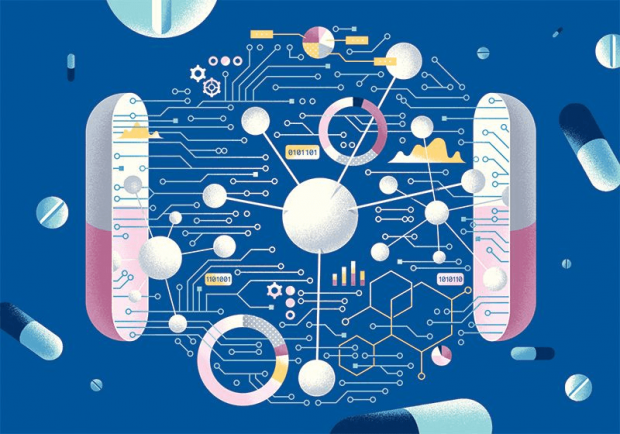
In terms of drug discovery projects, the scientists are in the process of building and pre-training neural nets which predict the binding energy of the candidate drug with the essential target proteins of the virus. It gives insights on how well the molecule binds to the target. In this way, we can get some good molecules which can then be subjected to biological assays. An RL framework has also been developed to synthesize new molecules. It uses a representation of molecules based on rigid fragments, which makes it really better because there are more abstract actions. It also makes these molecules more synthesizable. However, there are still a couple of challenges to address.
ML To The Rescue
The next lecture by Mihaela van der Schaar (John Humphrey Plummer Professor of Machine Learning, Artificial Intelligence, and Medicine at the University of Cambridge) titled ‘Using machine learning and PHE data to help hospitals cope with COVID-19,’ highlighted how the development of latest computing technologies will aid in building tools to help the healthcare workforce deal with this crisis. The goal of this collaborative research between ML-AIM lab and PHE (Public Health England) is to provide evidence that reliably assists healthcare professionals in making difficult decisions to save lives. It is using ML methods to forecast personalize risk, benefits, treatment, and resources for each patient such that individual hospitals can identify what type of resources are needed and when.
There are a lot of crucial decisions that doctors and clinicians have to make, such as when to transfer a patient to ICU, when should a person be put on a ventilator and for how long, when can they be shifted to general wards and when can they go home. The resources are getting increasingly limited, both in terms of ICU beds and ventilators. The aim is to accurately model the current demand and the forecast of subsequent demand. For this purpose, automated ML frameworks are being used. These are predicting the chances of a patient to die, be discharged, be admitted to the ICU or be put on a ventilator (when and for how long). This information will be useful for hospitals to do short term and long term future resource planning as well.
ML can be utilized to address several COVID-19 challenges such as estimating longitudinal trajectories of this disease, developing early warning systems, giving personalized treatment recommendations for each patient, informing policies, improving collaboration, managing uncertainty and expediting clinical trials, to name a few.
Looking At COVID-19 Through The Lens Of Data
This section comprised two small sessions from Fred Hamprecht (University of Heidelberg) and Devvret Rishi (Kaggle Initiative).
Basically, there is a need to better understand and tackle this pandemic on different time scales. In the short term, there is the question of the allocation of vital resources, optimal treatment strategies and making good epidemiological predictions to assist policymakers. On the other hand, the long term goal is the development of drugs and vaccines. Machine Learners will be able to make significant contributions to each of these stages and global collaborations among them (with science) are extensively needed to combat this viral illness.
Given the enthusiastic feedback of the participants, the next edition of this workshop was held on April 15th and the other one continued on April 22nd.
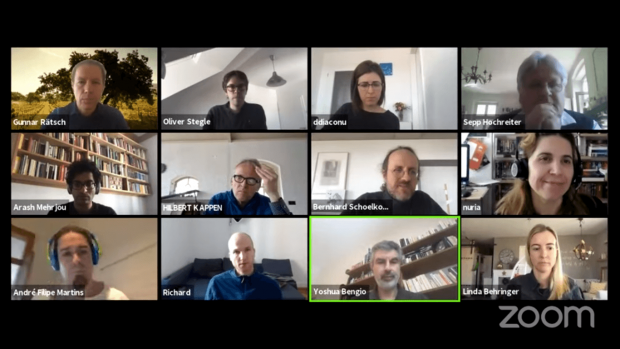