A data catalog is jet fuel for your machine learning and data analytics. Without it, you will have to spend a lot of time and effort on explaining your data, cleaning your data, and preparing your data for analysis. A data catalog provides context about your data set so that you can filter and search for the data you need. Data catalogs save you time by organizing data into similar categories. This enables you to find the right data set rather than opening data file after data file hunting for what you need. In the past, you had to work with data architects and other experts to create data catalogs. That’s starting to change due to some new tools.
The good news is that you do not have to create data catalogs manually. Amazon and Microsoft have created tools that make it easy to create data catalogs. With Amazon, you can use AWS Glue to create a data catalog without setting up your own extract, transform, and load (ETL) server. Alternatively, Microsoft offers the Azure Data Catalog which automatically creates metadata tags and makes it easy for your staff to add their annotations to the data.
This might sound a bit abstract so far. Why exactly would you want to put time and effort into creating a data catalog? Let’s illustrate that question by exploring one of the largest costs in financial services: fraud.
Using a data catalog to reduce fraud costs in financial services
Let’s say you run a bank and you are worried about credit card fraud. In financial services, fraud is a significant problem that requires a lot of staff, technology, and processes to keep at bay. LexisNexis research found that “In 2019 each dollar of fraud suffered by a retailer cost an incremental $3.13 in fraud-related expenses” Machine learning, supported by a quality data catalog, helps you to cut down your fraud management costs.
Stopping credit card fraud requires high-speed analytics. Since there are millions of credit card transactions around the world, it is not practical to have people review transactions individually. Instead, you need to leverage systems. A data catalog speeds up fraud detection by helping your machine learning focus on the right records. You can also use a data catalog to classify data for security protection and reduce the chance of misuse.
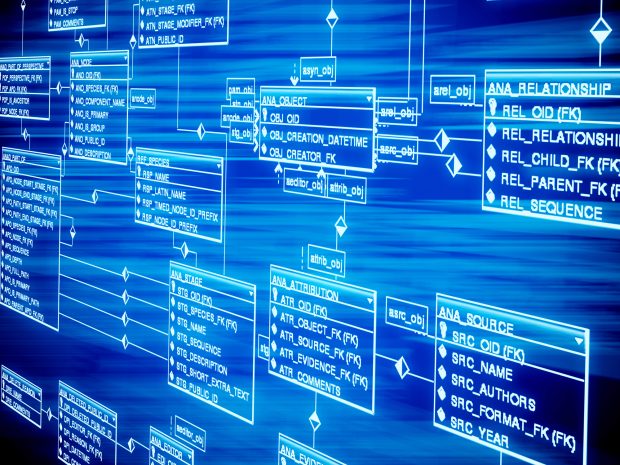
Five data catalog mistakes to avoid
Now that you see the value of a data catalog, there are a few common mistakes that are important to avoid.
- No Business Glossary. This resource defines the business context of the data. In the context of credit card fraud, a business glossary may define risk scores, credit scores, and standard abbreviations (e.g. probability of default or “PD).
- Lack of Automation. The best catalogs leverage automation techniques to connect to new data sources. If you lack this capability, your staff will have to spend more time manually connecting to new data sources.
- Failure To Manage Access and Permissions. A data catalog is an important key to understanding a large amount of your company’s data. Therefore, it is important to protect it like other sensitive data files. For example, apply the principle of least privilege so that few people have access to the resource.
- Lack of Independent Validation. Since your data catalog is a guide to machine learning and people, it is critical that it is accurate. That’s why we recommend having an independent review of the data catalog before it goes into production. This can be done by a different team in your technology department or by a third party consultant.
- Missing Tribal Knowledge. Think back to the last time you needed data for an executive report or business intelligence (BI) project. You probably asked one or two subject matter experts for help. They gave you tips on which datasets were the most reliable and useful. That type of data expertise is essential for machine learning success! That’s why we encourage you to capture this type of “tribal knowledge” in your data catalog.
Once you avoid these problems in your data catalog, it is time to plug this resource into your machine learning project.
Improving machine learning efficiency with data catalogs
In most machine learning projects, you need to feed a “training data” set to the machine to generate insights. For example, let’s say that you are considering offering a line of credit to a business. Before doing that, you want to put the borrower into a broader context of nearby companies and understand how many competitors they face. You could use the Yelp Dataset which includes over 200,000 businesses to start your machine learning. Alas, these external data sets will only take you so far.
For the best results, you want to feed your machine learning algorithm the most relevant data first. Using a data catalog, you might select businesses with similar size characteristics (e.g. number of square feet, number of employees). You might also only want to feed recently validated information to machine learning. That’s easy if your data catalog has a field like “Validation Date.” In this simple example, a few minutes of analysis mean you can extract a highly relevant data set for machine learning analysis. That means fewer false positives and effort to refine the model.
Data catalog use case
InTapp is an AI and ML solution provider to legal, accounting, and professional services firms across the nation. Data cataloging aids in legal research, accounting practice management and can lead to tremendous time savings, allowing employees (paralegals and junior staff) to work on higher-value tasks. InTapp vouched for the efficacy of data catalogs due to how they can help facilitate the cleaning, organization and querying of data. They believe in them so much that they have a little twist on them within their sector called CDS or Common Data Storage. It differs from “cataloguing” insofar as it is an actual data repository vs a table of contents showing where the data can be found.
“Our solutions and platform gather all the firm data into our CDS and then join the dots – connecting the data, connecting the users and creating are more efficient client lifecycle within the firm.” –Milan Bobde, Director of Product, InTapp
InTapp believes the benefit of data catalogs is that it creates a system of insight. It is the data aggregated, correlated and segmented according to the users’ need at any point in the client lifecycle. Recent events have spotlighted the need for this kind of access to specific data and specific moments. Rather than merely cataloging, Intapp delivers a connected data experience that creates greater efficiency in firms that sell their time.
From data catalogs to machine learning setup: Where to get data help
Your organization may already have business intelligence experts and analysts. However, they might be too busy with monthly and quarterly reports to explore machine learning. Asking them to take on the task of learning how to do machine learning analytics isn’t realistic right now. To get fast results without overwhelming your team, co-develop with top-ranked AI consulting and development agency Blue Orange Digital.
For more on AI and technology trends, see Josh Miramant, CEO of Blue Orange Digital’s data-driven solutions for Supply Chain, Healthcare Document Automation, and more.