A time-series analysis of underlying asset movement as a function of option trades, powered by the Facebook Prophet model.
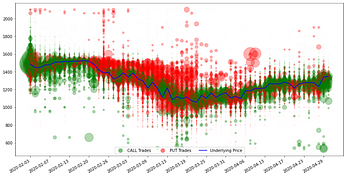
1. Introduction
1.1 Options & Their Use In Stock Forecasting
The traditional view of an option is as a derivative security, valued based on dependence on an underlying asset. Various mathematical models have been applied to determine the value of an option as a function of asset performance, while the inversion of this relationship has seen limited exploration. Many deride the notion that options can be used to accurately determine the future price of an underlier.
In recent months, the publicity around the options market has skyrocketed — the culmination of year after year of record-breaking trading volumes. This means that more information than ever before is publicly available. The data is there to be interpreted.
Values like the Put-Call Ratio (PCR) have been used as strong indicators of market sentiment, but their application has been very limited. The most common use of PCR is in “bands” — determining cutoff points to create ranges that can serve as indicators of whether the price of a stock will go up or down. While this is informative, it is a forecast lacking in many aspects, most notably the magnitude of change.
By analyzing large sums of data, we can move past the established status quo.
1.2 Data-Driven Discoveries
As readers of DataDrivenInvestor know, computer models allow users to analyze patterns in data that are otherwise inaccessible. While a human might not be able to summarize complex tabular data through conventional means like mathematical equations, a machine learning model can recognize trends that repeat themselves throughout the dataset.
In this analysis, we use the Facebook Prophet model for time-series analysis, to interpret the momentum of an asset indicated by option trading volume. It is known that option trades can be used as indicators of market sentiment and that the model can recognize trends similar to those found in the training data. Therefore, as patterns from the past begin to reemerge, the model should successfully estimate changes in stock price that will occur in the immediate future.

2. The Data
2.1 The Raw Data Set
For this analysis, I used options trading data for Alphabet Inc. (GOOG) from the year 2020. The high trading volume of GOOG options results in a significant dataset that is unlikely to be overly influenced by outliers within the data. While the amount of information available about GOOG option trades is vast, this analysis focuses on dollar volumes.
The sample period is centered around the COVID-19 pandemic induced market crash of 2020 — a significant data set with plenty of interpretable stock movement. Despite the highly volatile trends in the market during this period, the speculative nature of options contracts should provide a constant signal for short-term movement.
2.2 The Inputs
The inputs in this model were carefully selected not only to indicate the direction of a change in stock price, but also the magnitude. When determining the magnitude of a forecasted change, a model would essentially be evaluating the intensity of speculation. The most straightforward manner in which one can consider magnitude is simply in dollar value — the amount of money being used to purchase puts and calls.
Ultimately, the price of a stock moves because of financial pressures caused by buying and selling, so the aggregate price of purchased options should be a reliable indicator of movement. Therefore, instead of determining the value of an option via measures like volatility and strike, the model accepts the last purchase price at each strike as indicative of value.
The calculated input for the model is simply the sum of option volume times last price at each strike on any given day.

3. Using Time
3.1 Optimizing for Pattern Recognition
The relevance of put and call dollar volumes is relative, to standard quantities and to each other, for an expiration. As such, after being fed the raw data, the model calculates a modified put-call ratio (weighted by last price) to determine the likely direction of movement for the underlying asset.
Additionally, because the purpose of this model is momentum-based trading in relatively short day/week intervals, options with fast-approaching expiration dates are given additional weight. Simply put, options with distant expirations are not indicative of potential short-term trends of the model, and should be devalued when considering the short-term.
Therefore, when calculating the aggregate dollar volume on any given day, the data derived from each option is divided by days to expiration before being used to determine the sum.
3.2 Seasonality
The pattern recognition of the time-series forecast is based around the idea of seasonality — variations over specific intervals. For this model, I set the seasonality to a period of five days, in line with weekly option expirations. As such the model is best used on assets with high weekly options trading volumes.
With this information (especially when considered alongside the increased weightage provided to purchases near expiration) the model can more accurately determine movement based on reference points (expiration dates). The soft-reset in the forecast provided by expirations results in a model that is more robust in its ability to avoid overfitting based on a single outlier day.

4. Model Results
4.1 The Results
With the inputs determined and the seasonality configured, the model’s predictive capabilities can be tested using data from the past. As is evident in the graph shown above, the model was almost entirely successful in predicting the direction of price movement and was able to predict the most major magnitude changes.
Of particular note is the crash caused by the COVID-19 Pandemic, which began at the end of February 2020. Careful analysis of the results reveals that by using options trades, the model was able to effectively predict both the crash and the recovery two days before they actually occurred.

4.2 Modifying Inputs
Using different sets of inputs with the model revealed that shorter time frames result in better predictive capabilities for the model. As seen in the graph above, using the model over a four-month period instead of a year, resulting in outputs that were more accurate when compared to actual prices.
Overall, the model proved to be not only accurate but also robust, adapting to modified inputs and still delivering results. Ultimately, the concept behind the model is sound — that there are trends within options trading data that can indicate both the direction and magnitude of a stock price’s movement. Data science allows us to interpret them unlike ever before.
5. Conclusion
The ability of machine learning models to interpret trends in data far surpasses that of the human eye. This work proves that data science allows options trades to serve not only as an indicator of sentiment but also as a tool through which investors can determine the likely magnitude of a change in stock price.
This proof of concept, made possible because of modern modeling technology, has the potential to drive innovation surrounding the rising options sector. For years, investors have made trades based on implied volatility and the direction of movement indicated by options. Now, they can consider the extent of a potential price change as well, and invest accordingly.
As the inputs are end-of-day trading data, the model is evaluated based on its ability to predict next-day price. The success of this model indicates that consideration of real-time data could allow investors to predict intra-day fluctuations as well.
While it’s almost impossible to consistently predict the price of a stock in the distant future, using data science, one can determine momentum and make trades based on predictions for the immediate future. A deeper interpretation of option trades can become one new tool in the expanded toolbox of a data-driven investor.
An incredibly interesting article with a novel hypothesis. The author is indeed correct in saying that options are often dismissed as a price indicator, but the data shows quite a bit of potential. Looking forward to reading more.
A colleague shared this article with me earlier today and I must say, despite my skepticism, it was worth the read. While it should be noted that the article covers a relatively small sample, I do believe that that the strategy as a whole is promising.
I’ll definitely be keeping a closer eye on the options market in the future.
Global markets move on news and it can be seen in the advancement or the decline in the index futures as stocks trade around the world. The index futures are one indicator that offers important information as we approach that open.
The author is quite right, but you don’t have to stay up all night wondering where stocks might be when you get out of bed.
I’m a strong believer in momentum investing and the put-call ratio is yet another indicator to read trends from market data, it’s amazing how we get increasingly more data-points to quantify human emotions. However, the origin of market swings – for now – remains fear and greed. Human fear and greed. Whatever metric manages to capture that first will be suitable to use. The second layer of datapoints will then be the things that can be read from market data – which however requires the market to be open and decisions to be made already – and then robos and algos will have at it. Interesting read, thanks for sharing.