Misinformation has taken the world by storm, as it’s all around us. It’s in the form of posts filled with fake news, edited pictures depicting meanings differing from their original intentions, and now, even in the form of fake videos created using artificial intelligence (AI). All of this fake data can be created using AI, specifically by using Generative Adversarial Networks (GANs). GANs have been used to generate tons of fake content, such as creating writing similar to Shakespeare’s writing, to generating captions based on a photo.
However, the most concerning is definitely the generation of fake videos, also known as deep fakes. This article will provide a breakdown of how GANs work, go more in-depth with deep fakes, and some of the dangers concerning them.
The Generative Model – The Spider Spinning the Web of Lies
GANs consist of two different neural networks, the generative and discriminative models.
The generative model is the neural network that generates fake data. In order to do this, it’s fed lots of training data, and uses a reinforcement learning approach to train the model. The generative model is ‘rewarded’ if the discriminative model can’t discern the difference between real data and the generated data. The training of the model also focuses on the weaknesses in the model. For instance, if the model is being trained to recognize handwritten digits, and it isn’t able to distinguish one from seven, then the model will focus solely on trying to recognize the difference between those two numbers.
The model attempts to generate new data based on its learnings from the training set and tries to improve its method of generation until the generated data is indistinguishable from real data.
The Discriminative Model – Identifying the Difference Between the Truth and the Fabrication
The discriminative model tries to tell the difference between real data and the fake data generated by the generative model. For example, if the GAN is being trained to write similar to Shakespeare’s style of writing, the discriminative model will try to tell the difference between plays actually written by Shakespeare, and those fabricated by the generative model. Just like the generative model, the discriminative model is trained through a supervised learning approach. In order to tell the difference between the truth and the fabrication, the model estimates the probability of whether the data provided by the generative model is from training data set containing real data, or if it’s generated. Once the model has made its estimation, it outputs a number between 0 and 1 to relay whether the data it was given was real or fake.
The discriminative and generative models have a symbiotic relationship, which allows the generated data to become more and more realistic. The discriminative model provides the generative model with feedback on how to create more realistic data, and as the generative model improves, the discriminative model also improves at spotting the differences between real and fake data.
Deep Fakes – Crossing the Line with Fake Data?
As mentioned earlier, GANs can be used to create fake videos called deep fakes. Deep fakes modify/produce video content to depict something that never happened. For example, researchers at Washington University created deep fake displaying a fake Barack Obama giving a speech that he gave decades ago. Now, any audio can be mapped to the deep fake version of Obama, and it’ll look like visually realistic. The image below shows the synthetic version of Obama side-by-side with the real him. Isn’t it unnerving how similar they are?
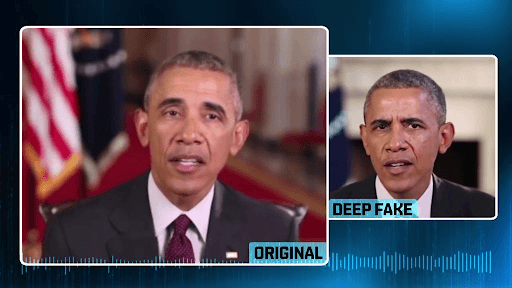
A Step-by-Step Guide to Generating Deep Fakes:
- The generative model is given a training set of videos similar to the desired output. For example, in order to create the deep fake of Barack Obama, 14 hours of footage was used so the generative model could learn to mimic the former President’s mouth movements.
- The generative model creates many fake video clips.
- Once a high-quality output is fabricated, the generative model starts feeding the clips to the discriminative model.
- The discriminative model determines whether the clip is real or not.
- Every time the discriminative model can identify a fake clip, it provides the generative model with feedback on how to improve future clips to make them seem more realistic.
Key Takeaways
- GANs can be used to generate many types of fake data.
- GANs consist of two neural networks, the generative and discriminative models.
- The generative model generates fake data.
- The discriminative model decides whether the image generated by the generative model is real or fake.
- The discriminative model provides feedback to the generative model on how to create more realistic data.
- Deep fakes modify/produce video content to depict something that never happened.
Data generated by GANs can be very difficult to spot, especially deep fakes. Deep fakes have already made their rounds on social media platforms such as Facebook and Twitter, and have proven how detrimental they can be by fueling the distribution of fake news. Some have even gone so far as to claim deep fakes will be the end of democracy, as fake political statements can be staged with this type of technology, resulting in devastating blows to political campaigns. Leave a comment down below regarding your opinion on deep fakes, and GANs in general. Do you believe data generated by GANs will only be harmful or are there positives to this technology as well?