How new technologies cannot avoid the same old problems.
Data is the new gold (or new oil – you pick).
Data-driven companies rule the world.
Data science is a game-changer.
AI is shaping the future.
There is no shortage of catchy slogans. Data analytics is sexy. Data lakes are the new hype. Data science is the new science. We hear a lot about leading companies increasing the pace of their Big Data and AI investments.
Now let’s put all the hype aside for the moment and look at the reality. With enormous investments in the last few years, how are these large companies doing in terms of successful implementations?
Let me take you back to Gartner’s Top Data and Analytics Predicts for 2019 by Andrew White. Consider these predictions made in January 2019:
…By 2022, 90% of corporate strategies will explicitly mention information as a critical enterprise asset and analytics as an essential competency
…Through 2020, 80% of AI projects will remain alchemy, run by wizards whose talents will not scale in the organization.
…Through 2022, only 20% of analytic insights will deliver business outcomes.
The desire of the majority of enterprises to become data-driven is evident.
But what about their capabilities? Why are we talking about “alchemy?” Isn’t that the opposite of science?
With all the money pouring into data analytics initiatives, how come the Gartner experts are predicting only a 20% success rate?
Now, let’s have a reality check with a report from NewVantage Partners, “Big Data and AI Executive Survey 2019.” The survey, completed by Chief Data Officers and various C-suite executives from major companies, confirms the burning desire to get value from big data and AI solutions:
…The pace of investment in Big Data and AI is increasing – 91.6% confirm the increase in pace.
… Executives report a greater urgency to invest in Big Data and AI initiatives – 87.8% confirming this urgency.
… Executives reinforced that they are (motivated by a fear of disruptive forces and competitors, with 75% of executives acknowledging this concern.
While the urgency is there, the reality is starkly different.
“An eye-opening 77.1% of executives report that business adoption of Big Data/AI initiatives remains a challenge for their organizations – an increase over 2018,” the same report states. As the report zeroes in on the business adoption challenge, it further expands on how the firms ranked themselves on transforming their businesses:
- 71.7% of firms report that they have yet to forge a data culture
- 69.0% of firms report that they have not created a data-driven culture
- 53.1% of firms state they are not yet treating data as a business asset
- 52.4% of firms claim that they are not competing on data and analytics.
Another event for AI industry leaders, Transform 2019, claims that 87% of data science projects never make it into production.
Now, it’s time for the (literally) million-dollar question. Why? What are the barriers? Where exactly are these initiatives failing? What is holding back the data-driven decision-making?
The summary in the NewVantage report is blunt:
Cultural challenges remain the biggest obstacle to business adoption… Executives cite multiple factors (organizational alignment, agility, resistance), with 95.0% stemming from cultural challenges (people and process), and only 5.0% relating to technology. If companies hope to transform, they must begin to address the cultural obstacles.
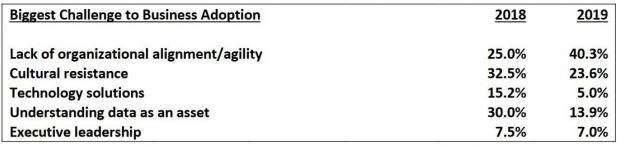
With the great expectations of game-changing results from analytics and big data implementations, the discussion about success factors (or lack thereof) has intensified.
As I re-read and analyzed reports, articles, and research from industry leaders and think tanks, I began to see a familiar pattern.
Most of the success factors and failure reasons for the analytics and big data projects are the same as the factors that impact any other business or digital transformation: humans, human behavior and biases.
For all the hype, incomprehensible technologies and the incredible rise of the boring statistician turned sexy data scientist, big data projects are still initiated and run by humans. And humans are fallible in predictable ways.
We have learned to recognize the impact of organizational factors on software development projects. I am firmly in the human factors camp, and reinforced the importance of the mindset on the success of business analysis in my book.
Whether the technology is old or cutting edge, the issues stay the same. Let’s do a quick recap – many of these points will be painfully familiar.
Not solving the right problem
Every investment must solve a business problem and align with the strategy.
Building a data lake because everybody is doing it is not a good enough of a reason. “Everybody is doing it” is not a good enough reason to build a data lake. Dabbling in AI to impress corporate buddies on a golf course is not going to impress anyone in the long term.
Sure, you can let your developers and data scientists play in a sandbox with the data – to learn and gain expertise. But if they continue to come up with great solutions that do not solve important business problems – then something is broken.
The data experts need to ask business stakeholders about the problems that need to be solved.
Data scientists can suggest potential solutions, but the problem statement and enterprise knowledge must come from business.
“No matter how good the team or how efficient the methodology, if we’re not solving the right problem, the project fails.” – Woody Williams
Check with business analysts about the importance of asking the right questions.
Asking the right analytics questions is just as crucial.
Hasty, unclear or absent business requirements
Business requirements are regularly cited as the major factor of project success (or failure).
According to the Standish Group’s well-known CHAOS report, project failure factors that can be attributed to requirements (lack of user input, incomplete or changing requirements, unclear objectives) add up to between 40-50%.
This is no different for analytics and big data. When projects are undertaken with the “Let’s build it and they will come” philosophy, the outcome is often a product without customers.
Every project must start with the business need, business problem, and the use case.
What will analytics be used for?
What decisions will be made using the predictions?
What needs to be measured and what are the business rules for measuring it?
Without asking the right questions, the analytics projects will become a sunk cost.
Projects are too large
Data lakes are becoming a place for companies to dump all their data. Even with raw, unprocessed data, a data lake for a large corporation may take years to build. This may amount to years of sunk investment without any return.
As the CHAOS report reminds us, the larger the project, the lower its chances of success. In the 2015 report, the researchers found that only 2% of the projects in the largest group (“grand”) have succeeded.
By using iterative development and starting small, we can greatly increase chances for success. According to the same CHAOS report, “agile projects have almost four times the success rate as waterfall projects.”
Business analytics and machine learning initiatives, due to their complexity and often-lacking qualified resources, are even more vulnerable to being buried under their own weight.
Starting small, working on a few real business problems, and learning from them before scaling up is the most sensible strategy for dipping one’s toes in a data lake.
“When an AI project is successful, it is often built on top of many failed data science experiments.”
– Santiago Giraldo, Cloudera, as quoted by Forbes.
While we are talking about scale, let’s not forget that big data does not exist without small data. For many business problems, using small data is quite sufficient, and much more manageable.
Business stakeholders are not ready
69% of NewVantage survey respondents stated that their companies have not built a data-driven culture. This tells us that their business stakeholders are not ready for incorporating data analytics into business processes and decision making.
What does readiness entail?
It starts with data literacy, requires business ownership and active participation in data governance, and needs an established level of trust in analytics and big data solutions.
The need for trust is yet another reason to start small. Learning and building success gradually will help gain trust and a level of comfort for business stakeholders.
If you start with the most complex problem your enterprise is facing, you risk not only the investment but the reputation of data-driven initiatives. If the ambitious big data undertaking fails, stakeholders’ distrust, coupled with wasted resources, will become a barrier for future analytics projects.
Corporate silos and lack of cross-departmental collaboration
If you have read this far, I don’t have to convince you that these issues will impair anything that an enterprise is trying to accomplish.
Business analysts struggle with this problem every day. They know all too well how silos, disparate agendas and lack of collaboration affect the analysis process and requirements (which will take us back to reason #2).
They also know how stakeholder resistance can block the progress of a project, often driven by the fear of being replaced by a system. This fear is only exacerbated when managers do not understand enough about the AI and the need for humans to monitor, train and improve the AI models.
“AI is not going to replace managers, but managers who use AI are going to replace those who don’t.”
– Deborah Leff, CTO for data science and AI at IBM, as quoted by VentureBeat
Optimizing the wrong process
In my business analyst days, I saw my fair share of projects that were supposed to “automate the current process.” If a business analyst does not question the current process, then the project risks cementing an old, suboptimal and overgrown in fluff process into the new application.
Every process needs to be captured, reviewed and analyzed for efficiency before automating. We must apply the same philosophy before introducing any predictive analytics and decision recommendations into business processes.
Insufficient training and change management
Cultural resistance can put brakes on any change. Without user adoption, the solution fails before it has a chance to prove its value. Without continuous smart communication, training and internal marketing, even a good solution may be doomed.
As we have seen in the figures above, more than 23% of analytics challenges have been attributed to cultural resistance.
Due to the complexity of advanced analytics and predictive modeling, the risk of distrust or misunderstanding of the solution is also high. Explicability of predictive models plays a big role. And the overall level of data literacy in the organization will determine how much effort is needed to foster user adoption.
This list of reasons is not exhaustive. There are additional factors at play that are specific to machine learning and AI technologies, from data quality and availability of qualified talent to the risk of introducing biases into machine learning algorithms.
If, however, we can remove these obstacles and learn to put our human biases aside – then, perhaps, the other challenges will not be so intimidating.
It’s all about people. About us.
Νatural sciences such as mathematics and physics are used to methodologically study and examine standard systems based on fundamental rules of nature. Therefore A.I and Machine learning when applied in business and economy, which belongs to the social sciences, would result in inconsistency.